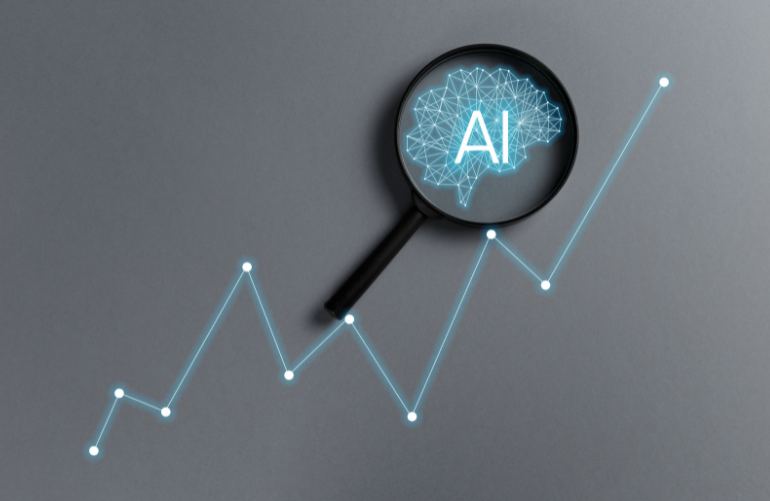
Intro to Bias in AI
AI in healthcare is becoming increasingly discussed due to its ability to enhance human intelligence. However, it’s important to discuss algorithm bias that reflects human biases found in care. Furthermore, it’s important to note that bias can exist not only in the algorithm but also in the predictions made by AI [1]. Unfortunately, bias in care causes marginalized communities to receive poor care and increases their mistrust of AI [1]. Therefore, it’s important to acknowledge any and all biases that exist in AI so that all population groups receive adequate healthcare services.
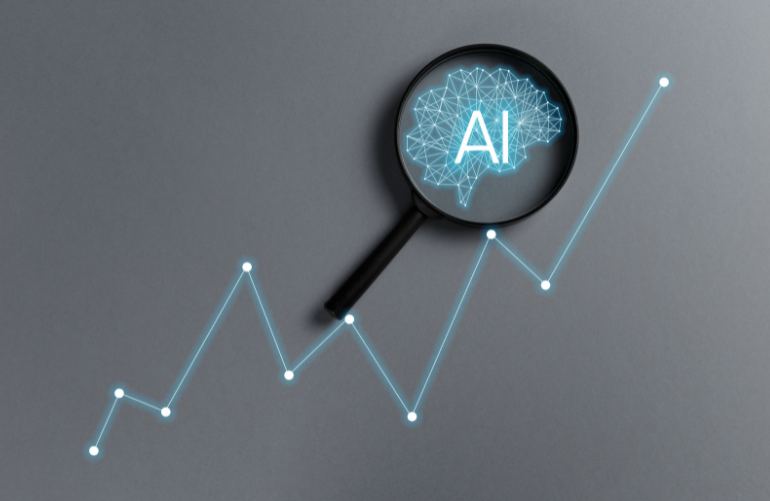
Bias in Training AI
One of the common ways that bias in training occurs is due to the lack of representation in training databases. For instance, research shows that Caucasians were found to make up around 80% of data [2]. This means that when a Caucasian person presents AI with their symptoms, they can get more accurate predictions. Furthermore, a study showed that the risk score performed ideally for Caucasian patients but not for African American patients [2]. This further proves that bias exists in data and that it will lead to inequalities in care for misrepresented populations.
Additionally, there was a case study that predicted the likelihood of women having a safe birth through vaginal delivery [3]. Unfortunately, the algorithm predicted that African American and Latino women were less likely to have successful vaginal births after previously having a C-section compared to white women [3]. This in turn led to doctors performing more C-sections on African American and Latino women [3]. It took years of additional work and changes to the algorithm before these biases were corrected [3]. Therefore, AI databases must be checked consistently and thoroughly to avoid unnecessary complications for marginalized populations.

Bias in Developers
Furthermore, developers who train AI may have inherent biases which are included in design choices. These design choices may make it harder for marginalized communities to be diagnosed properly. They may undermine certain symptoms and treatment plans, and overall result in biased outcomes. This can be corrected with a developmental team that is diverse so they may prevent unintentionally bringing bias into the algorithm and catch it when it occurs. Therefore one of the most important things that can be done to prevent bias in AI is to be aware of its presence in AI. Only then can it be addressed at every step.

Bias Beyond Race and Gender
It’s important to note that although commonly discussed topics of bias revolve around race and gender, it can also form in different areas. For instance, religious bias in AI must also be discussed. Different religious communities may face barriers that make it harder to access healthcare services. This means that data used to train models from these communities may be misrepresented and inaccurate. Unfortunately, this will lead to skewed data that makes biased predictions and worsens health disparities. Furthermore, implicit bias from developers towards certain religions may make AI interact differently with certain patients. Additionally, a lack of understanding of religious practices may result in AI making culturally insensitive recommendations that serve no purpose to patients and may worsen their trust in the healthcare system. Therefore, it’s important that AI does not hold religious bias in treatment choices and worsens already existing health disparities for marginalized groups.

Importance of Removing AI Bias
it’s becoming increasingly important that AI does not have a bias as healthcare technologies advance. This is because of how much AI is starting to be used both for diagnosing and for administrative tasks. Bias in AI models leads to unequal access to healthcare services. This provides increased disadvantages to already marginalized communities. Furthermore, patient safety is at risk due to incorrect or delayed diagnoses. Therefore, AI must make accurate and reliable predictions so that patients have better health outcomes. It’s also important that diverse communities and populations work with AI developers to test these algorithms for their accuracy [3]. Furthermore, algorithms should be released slowly so feedback can be thoroughly provided and corrected as needed [3].
Overall, removing bias in AI will help reduce health disparities and will improve health equity. Therefore, developers must focus on human-centered algorithms when developing AI. This means that disparities based on race, ethnicity, age, culture, religion, etc. are not amplified even more through the use of AI. Additionally, preventing bias in AI will improve trust, and provide more equitable and timely care to patients.
HITS
HITS provides management services and collaborates with clinicians in the development of health informatics. We provide tools that promote safe, timely, patient-centered, and equitable care. Our agency culture and mission facilitate customer and human-centered design. Additionally, we tailor software and project management support products to meet our customer’s needs. HITS also focuses on transforming health care by analyzing integrated medical solutions and evaluating information systems. Our goal is to enhance individual and population health outcomes, improve patient care, and strengthen the clinician-patient relationship.